Author: Dr. José Luis Mateos | Director of Digital Transformation at Honne Services
When one reviews the history of artificial intelligence (AI), one finds a period of emergence, like many other fields of knowledge, but also the so-called AI winters. These two winters correspond to periods in which economic support and investment from companies and governments declined, as a result of disappointment when the expected expectations were not obtained.
In this article we will analyze what caused the emergence of these two winters, how we managed to get out of both, and the reason why a third AI winter is not expected.
This last point is of crucial importance for companies, since understanding why there will not be a third winter and that, therefore, the future of AI is bright and promising, is vital for decision making and strategic planning in the companies.
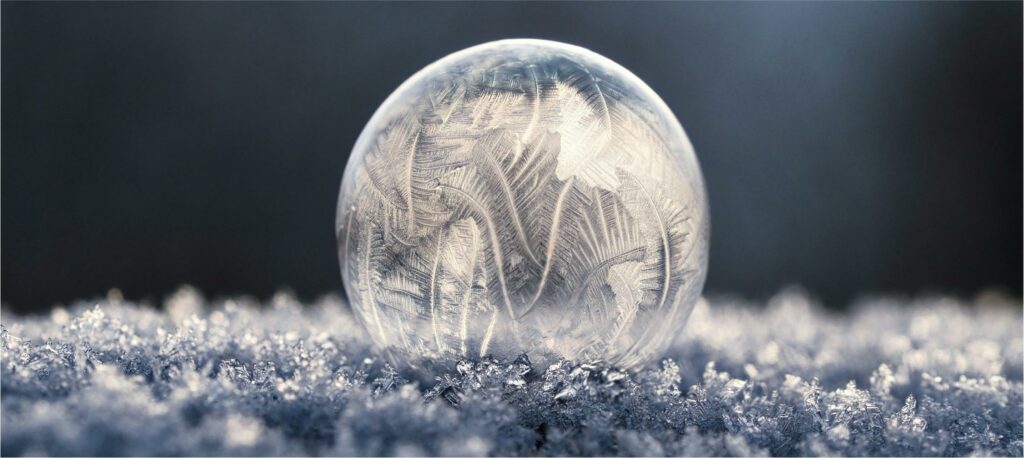
The two winters of AI
The two major artificial intelligence (AI) “winters” refer to periods during which enthusiasm, funding, and progress in the field of AI experienced notable declines. These periods were characterized by exaggerated expectations, followed by disappointment and significant cuts in funding.
First Winter of AI (1970s and early 1980s)
Causes:
1. Unrealistic Expectations: In the late 1960s, very high expectations had been generated about the capabilities of AI that could not be met. The researchers promised more than the technology of the time could deliver, particularly in terms of natural language understanding and machine translation.
2. Technological Limitations: The technology available at that time was insufficient to support the AI models and algorithms necessary to meet these expectations. Limitations in computing power and data storage were significant.
3. Reduced Funding: Disillusionment with the lack of progress led to a drastic reduction in funding from governments and private entities, especially notable in the United States and the United Kingdom, following critical publications such as the Lighthill report.
Overcoming:
Overcoming this first AI winter began in the late 80s, thanks to several factors:
– Focus on Specific Domains: AI began to be applied in more specific and controlled areas, such as expert systems, which demonstrated practical usefulness in fields such as medicine and geology.
– Advances in Hardware: Improvements in the processing and storage capacity of computers allowed us to address more complex problems.
– New Methodologies: The development of new techniques and approaches in AI, such as neural networks, which, despite entering a period of relative ostracism, laid the foundations for future advances.
Second Winter of AI (Late 80s and Early 90s)
Causes:
1. Failure of Expert Systems
Although expert systems found useful applications, they also faced significant limitations. They were expensive to maintain, difficult to update, and could not generalize their knowledge beyond their narrow application domains.
2. Limitations of Symbol Processing
The dominant approach to AI, based on symbol processing (or symbolic artificial intelligence), proved insufficient to capture the complexity of human reasoning and natural information processing.
3. Again, Reduced Funding
Renewed disappointment resulted in another round of cuts in investment, from both government and commercial sources.
Overcoming:
The recovery from the second AI winter occurred thanks to:
Internet boom
The expansion of the Internet in the 1990s exponentially increased the amount of data available, providing a rich source of information for training AI models.
Hardware Improvements
Improvements in processing power continued, especially with the arrival of GPUs for training neural networks, which facilitated the development of more sophisticated algorithms.
Advances in Machine Learning and Neural Networks
The resurgence of interest in neural networks, especially with the development of deep learning in the late 2000s and early 2010s, demonstrated capabilities that significantly surpassed previous approaches to AI.
These advances, along with renewed investment from both the public and private sectors, fueled a renaissance in the field of AI, leading it to the prominence and success it enjoys today. This renaissance has been marked by significant advances in natural language processing, computer vision, autonomous systems, and more, fueling optimism and investment in AI research and application across a wide range of sectors.
Overcoming the artificial intelligence (AI) winters was possible thanks to the contributions of leading researchers, academic institutions and technology companies. Collaboration between these diverse actors was instrumental in revitalizing the field, introducing technical innovations, new theoretical approaches, and practical applications that demonstrated the potential of AI.
Key people
Geoffrey Hinton, Yann LeCun and Yoshua Bengio
Known as the “godfathers of deep learning,” their research in neural networks and deep learning in the late 1980s and early 1990s laid the foundation for many of the current advances in AI.
Academic Institutions
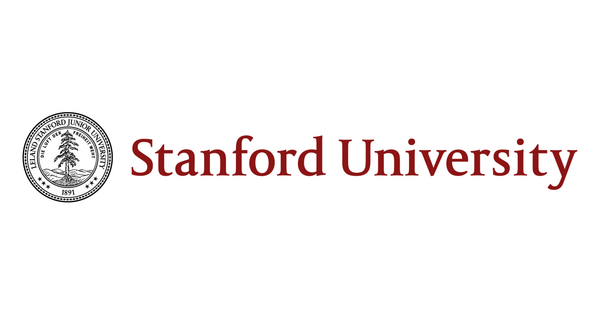
Stanford University: It was a powerhouse for AI research from its inception, playing a crucial role in the development of expert systems and the renaissance of neural networks.
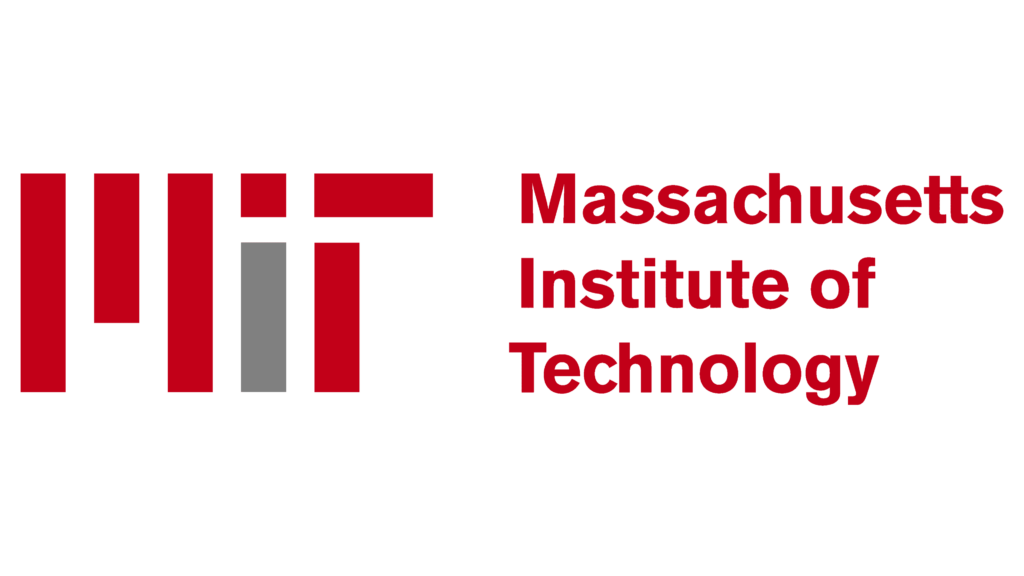
Massachusetts Institute of Technology (MIT): Contributed significantly to the advancement of AI through its AI laboratory, especially in the fields of robotics and natural language processing.
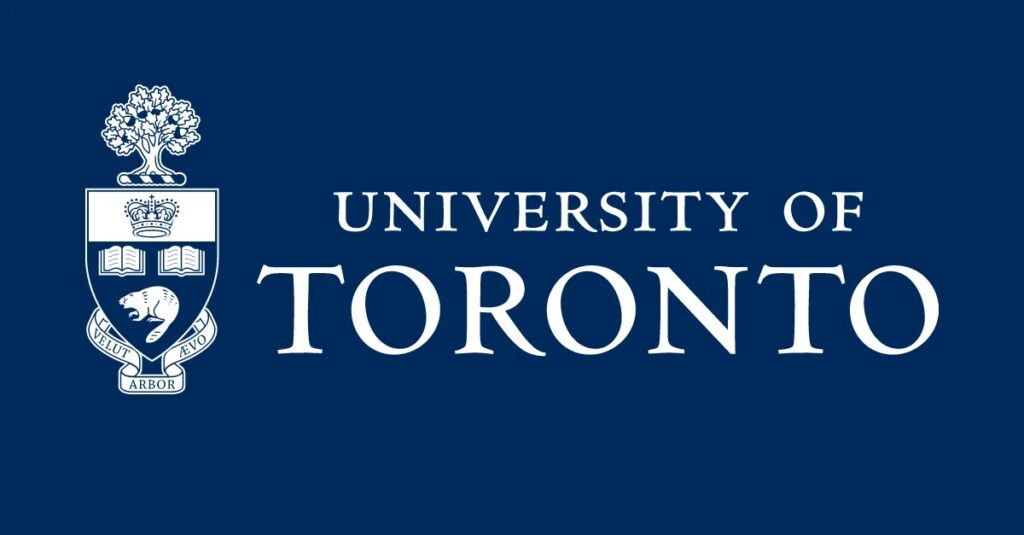
University of Toronto: Under the leadership of Geoffrey Hinton, it became a major center for deep learning research, contributing to the development of algorithms and techniques that advanced the field.
Technology Companies
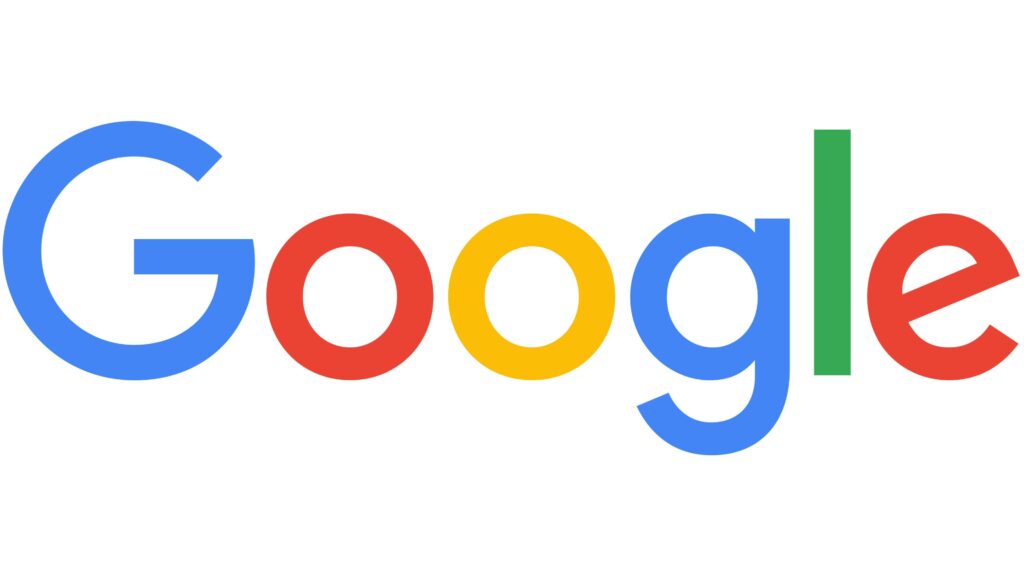
It has been a key player in promoting deep learning, especially through acquisitions such as DeepMind, whose research in reinforcement learning and other areas has driven significant advances in AI.
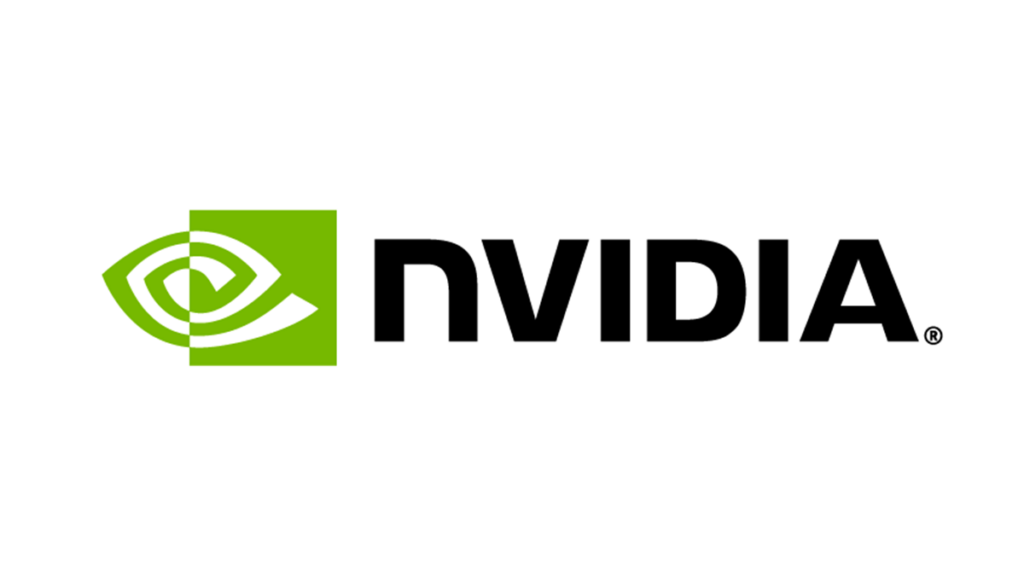
Although originally focused on computer graphics, NVIDIA has become instrumental in the advancement of AI by developing GPUs that significantly accelerate the training of neural networks.
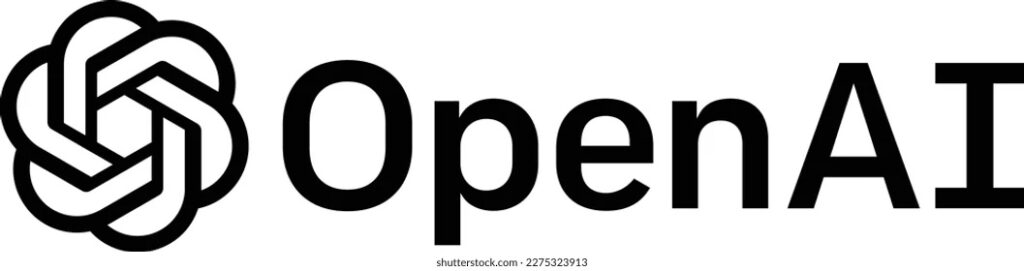
With the emergence of Transformers in 2017, a boom began in the study of natural language processing (NLP), which gave rise to large language models (LLMs) and generative AI with Generative Pretrained Transformers (GPT), with its famous chatGPT at the end of 2022.
Why won't a third AI winter happen?
To understand why the emergence of a third winter of AI will not occur, it is essential to take into account the existence of what I callthe virtuous shortlist:1) big data, 2) advanced hardware and 3) software and algorithms.
To expand and deepen the content on the crucial aspects of artificial intelligence (AI) – big data, advanced hardware, and powerful software and algorithms – it is essential to break down and further detail each of these three components of the virtuous triple, their interconnections, and how together they are accelerating progress in the field of AI.
1) Big Data: The Fuel of AI
The Rise of Data
In the current era, characterized by mass digitization, data is generated on an unprecedented scale. This phenomenon is driven by the proliferation of internet-connected devices, social media platforms, enterprise systems, and IoT sensors, producing a diversity of data that includes text, images, video, and sensor signals. These vast data sets, known asbig data, are the fuel that fuels advances in AI, providing the raw materials necessary to train machine learning and deep learning models.
Processing and Analysis Technologies
To transform these enormous volumes of data into useful information for AI, data processing and analysis technologies have been developed and perfected. Various tools and cloud technology facilitate distributed data processing, allowing large volumes of information to be handled efficiently. These systems use clusters of computers to process and analyze data in parallel, significantly reducing the time needed to obtain valuable information.
Challenges and Solutions in Managing Big Data
Managing massive data involves facing challenges related to its volume, variety, and speed. Integrating, cleaning, and preparing data from heterogeneous sources are critical tasks that require advanced algorithms and data analytics and machine learning techniques to ensure that the data is ready for analysis. Furthermore, efficient storage of this data poses challenges in terms of infrastructure and accessibility, driving the development of cloud storage and database solutions that offer scalability and flexibility.
2) Advanced Hardware: The Power Behind AI
Evolution of AI Processors
Progress in the field of AI has been paralleled by the development of specialized hardware that can meet its intensive computational demands. Graphics processors (GPUs) have evolved from their use in video games and graphic applications to become pillars for training AI models, thanks to their ability to perform large-scale parallel calculations. Similarly, tensor processing units (TPUs) have been specifically designed to accelerate machine learning workloads, offering significant improvements in speed and power efficiency.
Impact on Innovation and Development
Access to more powerful and faster hardware has catalyzed innovation in AI, allowing experimentation with more complex models and the exploration of new frontiers in research. This advance has facilitated the development of applications that were previously considered unfeasible, such as autonomous vehicles, advanced recommendation systems, and intelligent virtual assistants. The continued miniaturization and improvement in efficiency of these processors also promises to make AI more accessible and sustainable, paving the way for its integration into mobile devices and embedded systems.
3) Software and Algorithms: The Intelligence of AI
Going deeper into Deep Learning
Deep learning, a technique that uses deep neural networks to model complex abstractions in large volumes of data, has been fundamental to recent successes in AI. These networks, which mimic the structure and function of the human brain, have proven to be exceptionally good at tasks such as pattern recognition and classification. Advances in this area have been made possible by the availability of large data sets and the computational power necessary to train these networks, resulting in notable progress in computer vision, natural language processing, and other fields.
Innovations in Generative AI
Generative AI, including technologies such as Transformers models, have opened up new possibilities in the creation of synthetic digital content. These models can not only generate images, text, videos and sounds, indistinguishable from those created by humans, but they can also be used to design drugs, create art, and simulate complex scenarios for training and analysis. The ability of these models to learn and generate new patterns from existing data is creatively transforming numerous industries, from entertainment to biotechnology.
Challenges in Algorithm Development
Despite advances, the development of AI algorithms still faces significant challenges, including the need to improve computational efficiency, reduce data requirements for training, and increase the interpretability of models. Research into techniques such as transfer learning, semi-supervised learning, and reinforcement learning points toward solutions that can make AI models more robust, versatile, and understandable.
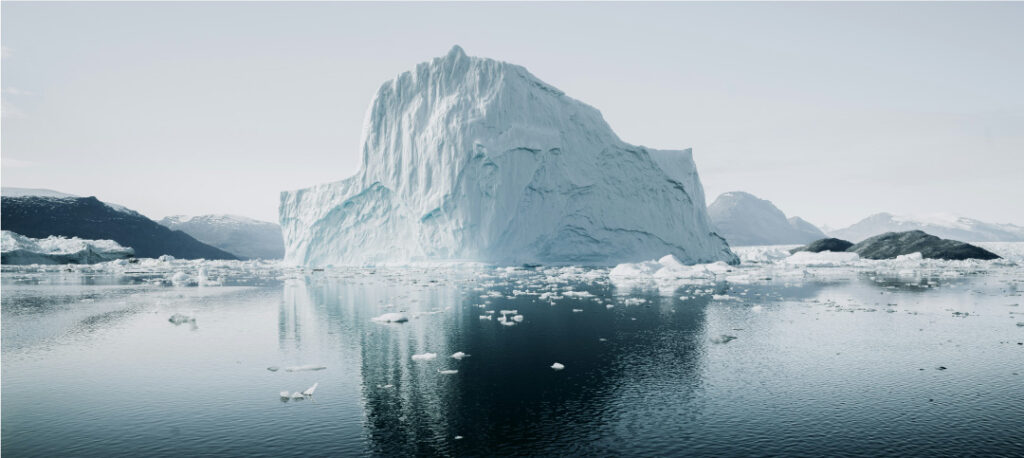
The interplay between big data, advanced hardware, and software and algorithms is driving an era of unprecedented innovation in the field of AI. As these elements continue to evolve in alignment, the possibilities for AI applications expand, promising significant transformations in virtually every sector of society.
The message for companies is this: since for the first time we have this virtuous trio aligned, it is clear that a third winter of AI will not emerge. On the contrary, a bright future is foreseen. Therefore, we must join this revolution. This is just the beginning: the tip of the iceberg.
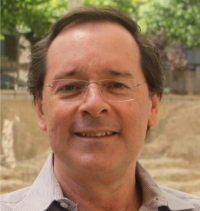
Dr. José Luis Mateos Trigos, Mexican physicist, PhD in Sciences (Physics) from UNAM, post-doctorate at Northeastern University. Researcher, research coordinator, award-winning author and science communicator. Director of Digital Transformation at Honne Services.